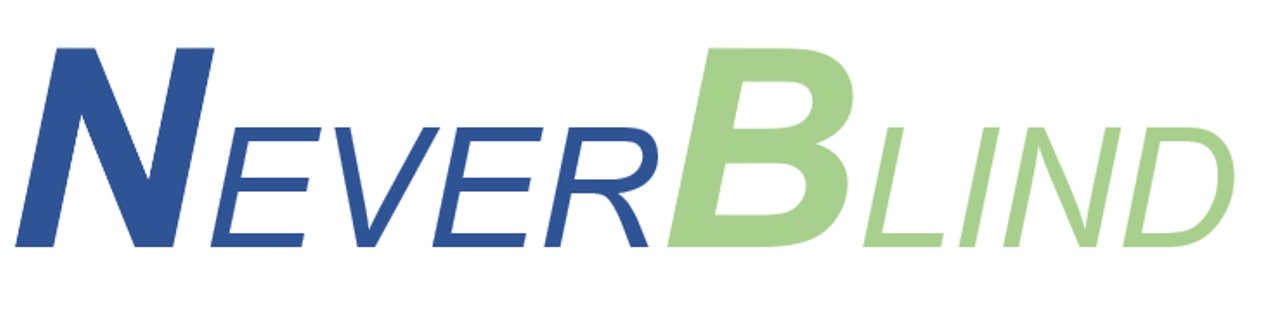
Problem statement
Diabetic Retinopathy (DR) is a neurovascular complication of Type 1&2 Diabetic patients that leads to complete vision loss. DR is the major cause of preventable blindness in working-age adults. Globally, DR is the fifth cause of blindness and visual impairment affecting 3-4% of Europeans. Moreover, DR affects 30% of diabetic patients and it is estimated that 280 million people are blind by diabetes in the world.
When detected on time, the risk of DR can be reduced by 95% but the disease is asymptomatic in its early stages and the risk of developing DR varies among patients. Diabetic Ophthalmology Associations recommend regular screening, while this can be done through telemedicine, most patients are still screened at ophthalmology units.
There are no standardized screening programs across Europe and the implemented solutions at the national level are notably expensive, time-consuming, and not fully adequate for the patient’s needs. Besides, existing healthcare systems are inefficient in detecting DR due to: 1) A high number of diabetic patients causing an excess of patients per available mydriatic camera; 2) Most patients are being screened at the ophthalmology units, even though there is the option of telemedicine with non-mydriatic fundus cameras; 3) This screening is more challenging outside urban areas (29% of Europeans), where ophthalmologist units containing non-mydriatic cameras are located.
UP2SMART platform for eye healthcare
UP2SMART developed a platform for early detection and diagnosis of DR combining artificial intelligence (AI) models. It includes two AI-based systems: MIRA and RETIPROGRAM. Mira is a fully automated eye fundus image reading system while RETIPROGRAM is a diagnostic assistance tool that uses AI techniques to model electronic health record data (EHR) of the population to predict the risk of developing DR.
UP2SMART’ platform can supply a real-time response that allows the health centre physician to make a decision for the subsequent steps in the treatment of a patient if it is required. Either the next check-up or diagnosis by a specialist in the ophthalmologist's office in the hospital in the event that the platform had found something possibly abnormal to be assessed by the specialist.
UP2SMART’ platform can improve the efficiency of DR screening, the productivity of healthcare systems and patient satisfaction. It mitigates the limitations of DR screening considering both the economic costs and patient outcomes, as follows:
- Help optimize scheduling indication, priority, revision and prioritization of diagnostic procedures according to pathology and clinical symptoms.
- Help non-eye specialised healthcare professionals (primary care doctors) in stratifying DR patients.
- Provide a personalized screening that will improve patient outcomes and satisfaction.
- Improve interaction between main hospitals and primary care centres to provide better and timely support and decrease the burden on families.
- Help reduce the number of patients reaching the later stages of DR and decrease treatment costs.
Diabetic Retinopathy (DR) is a chronic disease and one of the main causes of blindness and visual impairment for diabetic patients. Diabetic retinopathy is one of the most common morbidities associated with diabetes mellitus (DM). Normally, the appropriate control of the disease requires the implementation of expensive screening and following up programs. Thus, RETIPROGRAM and MIRA systems aim at helping doctors to identify and follow up patients with DR using artificial intelligence (AI) based tools extensively studied in a multidisciplinary research that has included ophthalmologist experts in retina.
RETIPROGRAM: A clinical decision support system for Diabetic Retinopathy early detection
This program is a Clinical Decision Support System that helps to discriminate between diabetic people that may develop Diabetic Retinopathy and others who do not have any risk.
The computer program is focused on people with Type-2 diabetes. It used 9 input risk factors that can be obtained from the Electronic Health Record of the patient, or introduced by a physician. Then, the system uses a set of fuzzy classification rules and a procedure of aggregation to determine the degree of risk of developing Diabetic Retinopathy.
The users of this system are doctors (family physicians or ophthalmologists), who receive a prediction of DR risk together with a degree of confidence for the result. Given that information, the system is able to calculate the most appropriate date for the next control visit of each patient.
MIRA System: Classifier of eye fundus images in to detect the degree of Diabetic Retinopathy
Preventive screening of the eye fundus is a usual technique to detect Diabetic Retinopathy disease (DR). Automatic tools for image processing and classification can facilitate the hard work of manual labelling of this kind of images, which requires high expertise and a lot of time from expert doctors.
An eye with DR may present some of the following lesions: microaneurysms, exudates, hemorrhages. The number, location and level permits to classify the disease in different stages. The computer System MIRA receives an eye fundus image and classifies the image into 4 categories: No DR, Mild DR, Moderate DR and Severe DR. In addition, in the preliminary stage permits to filter non-valid images, which can be images that do not correspond to an eye, or images with poor quality that should not be analysed. This tool avoids the classification in a DR level of images that are wrong.
The tool has been constructed using Deep Learning techniques for training 3 different models using a data set of labelled images. Ophthalmologists experts in retina have labelled and validated the images to assure the best performance in the system.
This system is not for use of patients, it is a support tool for doctors, who can check if the prediction given by MIRA really corresponds to what is observed in the image. In that sense, MIRA can be especially useful to avoid False Negatives, because the automatic classification system is able to detect very small lesions that are hardly visible by human inspection.
SYSTEM DESCRIPTION
In this section it is described how to use the MIRA system to automatically analyse fundus images of eyes in order to detect if a person has Diabetic Retinopathy (DR) or not. In case the system identifies the analysed eye fundus image has DR, the system classifies the level of DR among Mild DR, Moderate DR and Severe DR.